Deep Learning Enabled Nanophotonic Design via Finite Element Simulation
Metamaterials and metasurfaces are emerging technologies in the field of nanophotonics. They can enable the engineering of arbitrary optical response based on their nano structure. The desired effective optical response is quantified by amplitude and phase of the light reflected and transmitted from the metasurface. Using this approach there have been demonstrations of exotic optical phenomena such as modified Snell's law, Pancharatnam-Berry phase and Photonic Spin Hall effect.
However, increased functionality requires complex design of metasurfaces for which iterative computational simulations are required, raising the computational cost for forward design. An efficient approach for carrying out metasurface design for a given functionality is a data-driven approach. This approach entails calculation of multiple design parameters and functionalities of metasurfaces which are listed as a dataset for training a neural network, which could subsequently be used for optimal inverse design for metasufaces. Generative adversarial neural networks (GANs) is a data driven-machine learning model, which can learn the patterns and underlying correlation between metasurface design and their optical response. Hence with availability of highly optimized dataset, substituting GANs for simulations could reduce the computational time and have unconstrained metasurface structure design.
Here we study and optimize the design of metasurface as a chiroptical beam splitter demonstrating photonic spin hall effect(PSHE) for sequential separation of left circular polarization (LCP) and right circular polarization (RCP).The proposed beam splitter design for on-demand power efficiency with wavelength selectivity enables a platform for chiral sensing, which is relevant for biological applications. We simulate different geometrical design parameters of the beam splitter -- an array of gap-plasmon based nanostructure which on illumination with a light beam shows PSHE due to rapid gradient of phase on the metasurface. The optimization is done in near infrared region(NIR) and extended to optical for high power efficiency in the reflected LCP and RCP wave.
The design and optimization of the proposed nanostructure is carried out through COMSOL Multiphysics® using RF module with electromagnetic waves,frequency domain study.The dataset generation for different design parameters is done using COMSOL Multiphysics® via LiveLink™ for MATLAB®
LiveLink™ for MATLAB®
, where a random parameter generation algorithm is used for creating a design parameter space for different desired optical properties. This dataset is then used for training the deep neural network for inverse design of the metasurface.
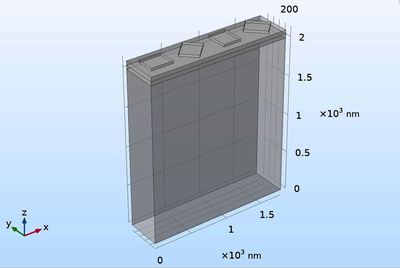
Téléchargement
- COMSOL_Bangalore_2019_AbhishekMall.pdf - 0.73MB
- Abhishek_Mall.pdf - 0.62MB